|
|
|

|
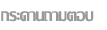
|
|
Prediction Of Cryptocurrency’s Price Utilizing Ensemble Machine Learning Algorithms
โดย :
Hayley เมื่อวันที่ : อังคาร ที่ 21 เดือน มกราคม พ.ศ.2568
|
|
|
<p>A cryptocurrency is a cryptographically secured digital foreign money distributed on a decentralized network utilizing blockchain know-how, <a href="https://blockchainreporter.net/daily-prediction/">coin valuation metrics</a> making them anonymous and untraceable. The advent of cryptocurrencies and blockchain know-how has sparked a revolutionary shift within the monetary sector (Kayani, 2023; Kayani and Hasan, 2024).</p><p>Cryptocurrencies provide substantial returns on investments besides possessing a better danger factor. A classic example can be the Bitcoin index (in EUR), which stands at a 108.27% compound annual growth price and an ordinary deviation of 156.99% between 2011 and 2024. Figure 1 exhibits the abnormal spike in Bitcoin return during 2021, with significantly increased fluctuations indicating heightened volatility. This calls for accurate forecasting models to fight the large fluctuations in non-stationary cryptocurrencies (Bouteska et al., 2024). Prediction of cryptocurrency prices can be important for a number of different reasons, similar to constructing trading strategies, risk management, price discovery, market sentiment evaluation and business functions.</p><p>Fundamentalists and chartists depend on worth prediction to make an informed resolution. Chartists in search of value patterns and developments can refine their technical analysis based mostly on forecasting. While fundamentalist’s major focus remains on long-time period value assessment, the model’s predictions may probably function a further data point for contemplating brief-time period market sentiment which may affect their investment decisions (Soltani et al., 2023).</p><p>The early-stage researchers employed statistical and econometrics models to forecast the worth of cryptocurrencies. To foretell Bitcoin’s quick-term prices, the autoregressive integrated moving common (ARIMA) mannequin was applied by Wirawan et al. (2019). The progressive progress in machine learning (ML) additionally motivated researchers to check the forecasting accuracy of various models. Lyu (2022) in contrast the accuracy of varied ML models and located gradient boosting (GB) to be the most efficient in predicting most major cryptocurrencies.</p><p>Recent research on forecasting started making use of deep learning (DL) and hybrid fashions, combining classical models such as the ARIMA model and synthetic neural networks (Suhartono et al., 2017). Li et al. (2022) discovered that the hybrid model improved the forecasting accuracy of overseas currency and demonstrated that the hybrid data decomposition model outperforms econometric, ML and deep-studying models. Chen (2023) predicted the Bitcoin worth of the following day and located that random forest (RF) offers higher accuracy than lengthy brief-term memory (LSTM). An extensive literature assessment revealed restricted research utilizing ensemble strategies to foretell the costs of a number of cryptocurrencies and hyper-tune the parameters to improve forecasting accuracy (Derbentseva et al., 2021).</p><p>Furthermore, the present literature predominantly focuses totally on Bitcoin. Empirical analysis is important to test the forecasting models in a broader range of cryptocurrencies that show diverse worth dynamics and volatility, which have advanced over the previous few years (Piryonesi and El-Diraby, 2020). ML has the ability to establish complicated patterns in worth movements and overcome the restrictions of traditional fashions to enhance forecasting accuracy. In contrast, conventional fashions like ARIMA could exhibit reliability in some cases (Nakano et al., 2018). Ensemble mannequin combine the output from multiple fashions, hyper-tune the parameters and enhance the forecasting accuracy. A scientific, three-stage method is adopted to create an ensemble mannequin for predicting cryptocurrency prices. First, a dataset of historical prices of the 15 cryptocurrencies chosen based mostly on their market capitalization. Then, the base models, random forest regressor (RFR), gradient boosting regressor (GBR), assist vector regression (SVR) and MLP regressor, are skilled on the coaching set, optimizing their hyper-parameters. With the bottom models skilled, predictions on the check set are made using every model. Finally, these particular person predictions are combined utilizing stacking to create the ensemble model. Root imply squared error (RMSE), imply squared error (MSE), mean absolute error (MAE) and R2 are used to evaluate the ensemble model’s efficiency on the 15 cryptocurrencies.</p><p>The study addresses the vital gaps in the existing literature. First, the existing research have explored the statistical or ML models in silos. The traditional statistical models fail to seize the non-linear and rapid price motion of cryptocurrencies. Further it carries limitations corresponding to hyperparameter tuning and lacks computational effectivity. The standalone ML models suffer from issues comparable to overfitting or underfitting adversely affecting the forecasting accuracy. This research bridges the gap by making use of a robust ensemble machine leveraging the potential of ML to enhance forecasting accuracy. Further the present studies on forecasting, focus totally on a single cryptocurrency, Bitcoin in general and few others like Ethereum. The broader cryptocurrency market remains largely unresearched. The study provides a methodological framework to empirically take a look at the forecasting accuracy of ensemble model on a broader set of cryptocurrencies. Ensemble fashions are flexible in capturing non-linear relationships, complex patterns in the data and provide superior forecasting performance as compared to the standalone fashions. This paper contributes to the existing theories on predictive analytics, providing empirical proof by creating an ensemble ML model. As well as, it contributes to the body of knowledge on how you can leverage bias-variance trade-offs in forecasting. To fill the analysis hole, the study goals to handle the following questions: (1) Tips on how to develop an ensemble model to precisely predict the prices of multiple cryptocurrencies? (2) Does the ensemble model outperform the statistical and econometric mannequin in enhancing forecasting accuracy? (3) Does the ensemble model present a better estimate of future worth on a broader set of multiple cryptocurrencies that differ in return and volatility dynamics?</p><p>The rest of the paper is structured as follows. A short literature evaluation is detailed in Section 2, outlining the earlier papers that used numerous fashions to foretell the price of cryptocurrencies. Section 3 explores the methodology and the ensemble prediction approach of this research, whereas Section four presents the empirical findings, together with a comparability of the proposed ensemble technique with econometric and ML models. The paper concludes with Section 5, offering avenues for future analysis in forecasting.</p>
เข้าชม : 49
|
|
กำลังแสดงหน้าที่ 1/0 ->
<<
1
>>
|
|
|